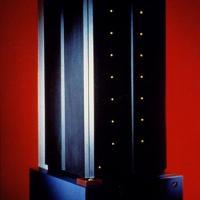
How Your Chess Program Defeats You, Part 2
In Part 1 of this blog, I explained how a chess program plays the opening, and I also outlined the Minimax procedure that is used to play the middle game. This blog continues the discussion by revealing the primary difficulty faced by a computer chess program, and how that difficulty is overcome. As before, we will refer to our nefarious computer opponent as the satanic “Chessifer”. Also as in Part 1, you need no background in computer science to understand this discussion.
In Part 1 we saw how Chessifer constructs trees representing the game from the current position. Chessifer can do this extremely fast. The IBM chess computer that defeated Garry Kasparov in 1997 could search roughly 200 million positions per second, although we must keep in mind that this was a special-purpose machine constructed solely to play chess.
Lest you fear that Chessifer is omniscient and/or omnipotent, he is not. I said in Part 1 that he computes “as much of the chess tree as he can.” He cannot compute all of it because the tree is too big. It contains more nodes than there are electrons in the universe, and this presents Chessifer with his first major hurdle.
He would like to compute as far ahead as possible, but he does not want to waste time following branches of the tree that are stupid, wasting his time. Fortunately for Chessifer (not you), there is a way to identify parts of the game tree that are useless to explore, allowing Chessifer to spend his time in more promising parts of the tree.
Healthy Trees Must be Pruned
There is a procedure called alpha-beta pruning that can determine when exploring certain portions of the game tree is not useful. Consider Figure 1.

In Part 1 I said that Chessifer first creates a partial game tree and then applies Minimax to assign values to all the resulting positions. That was an oversimplification. Actually Chessifer normally interleaves building the game tree with Minimax. That is the situation in Figure 1, where there may be many more branches under Position G, but before exploring those, Chessifer is going to apply Minimax to Position B. Since from Position B it is your move, Chessifer uses the Min rule, previously discussed, and assigns 27 to Position B.
This now raises an interesting question. Should Chessifer continue expanding the game tree at Position G? Notice that Eval has already given a value of 18 to Position F. What will happen when Minimax is ready to give a value to Position C? Since C is a Min Position its value will be 18 or less. And since that cannot be larger than the value 27 at Position B, the value at A can be assigned right now from B, and Position G can be ignored altogether. If there are a large number of positions under G, this could result in a significant savings of processing time.
The example in Figure 1 is an instance of “alpha” pruning. Figure 2 shows the related “beta” pruning.

Consider when it is your move at Position B. Obviously, you would avoid moving to E since Chessifer could then go to Position F with a value of 62. You would be better off moving instead to D, which has a value to Chessifer of only 47. Thus, 47 can be immediately assigned to Position B, and there is no need at all to expand the tree from Position G or perform any evaluations on those positions. Once again there could be a great many positions that are avoided, saving a tremendous amount of processing.
In the End is the Beginning
In Part 1 I explained how Chessifer uses a very large table to play the opening, rather than performing tree search. The same basic idea is used to play the end game, although the end game can be played even better than the opening. That is, the opening is based on current chess theory, as it is called, which is simply the collective wisdom of chess masters around the globe and which is collected into large reference works such as ECO, MCO-14, and others. But just as medical wisdom changes over time, so does chess wisdom, which is why these works are periodically updated.
But endgame databases are different in that, within bounds, they encode perfect play. By perfect play, I mean just that. If there is a win, the end game database can tell Chessifer how to get it. If the best that he can do is draw, then Chessifer will draw.
Endgame databases are created by what is called retrograde analysis. An ending position, either a win or a draw, is investigated backwards by finding all moves that can result in that end position, and then all moves that lead to those pre-final positions are found, and so forth. Such perfect end game databases have been constructed for all endings with 6 or fewer pieces and pawns. You can even download some end game databases from the web, if you search on “endgame tablebase”.
Conclusions
There is much more than Minimax and Alpha-Beta pruning that goes into a full-featured chess engine, but these are the essentials of what your computer is doing when it humbles you repeatedly. Other procedures and issues for the computer include such things as iterative deepening, the horizon effect, node ordering and critical nodes, the killer heuristic and more. If these first two parts receive sufficient interest, then I may write a Part 3 to cover additional concepts.
And finally, I would be remiss if I did not include an example of a chess program beating a human player. For this, I will present the first game where a computer made history by defeating a world chess champion. The game Deep Blue (pictured at the top of this blog) vs. Kasparov, 1996 marked a monumental achievement in the development of computer chess programs.